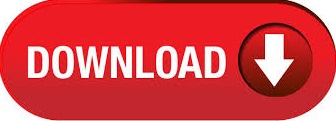
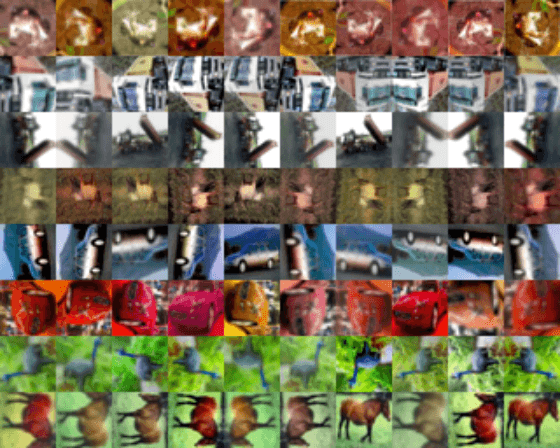
In the image, a comparison is given by some examples of EEG produced by the GPT-2 model and a human brain.Ī common approach is to generate synthetic signals by re-arranging components of real data. It was also noted that statistical machine learning models trained on the synthetic domain could classify the human data, and vice versa. In this study, it was noted that recognition was improved via data augmentation. It has also been noted that OpenAI's GPT-2 model is capable of learning from, and generating synthetic biological signals such as EEG and EMG. Ī comparison of GPT-2 generated EEG signals (left) and real human brainwaves (right) across "Concentrating", "Relaxed", and "Neutral" mental state classes. explored the idea of using Deep Convolutional Neural Networks for EEG-Based Emotion Recognition, results show that emotion recognition was improved when data augmentation was used. The approaches are also important in electroencephalography (brainwaves). noted that it is possible to use a Generative adversarial network (in particular, a DCGAN) to perform style transfer in order to generate synthetic electromyographic signals that corresponded to those exhibited by sufferers of Parkinson's Disease. Data scarcity is notable in signal processing problems such as for Parkinson's Disease Electromyography signals, which are difficult to source - Zanini, et al. The applications of robotic control and augmentation in disabled and able-bodied subjects still rely mainly on subject-specific analyses. Synthetic data augmentation is of paramount importance for machine learning classification, particularly for biological data, which tend to be high dimensional and scarce. Residual or block bootstrap can be used for time series augmentation. Additionally, image recognition algorithms show improvement when transferring from images rendered in virtual environments to real-world data. Another solution is the sourcing of entirely new, synthetic images through various techniques, for example the use of generative adversarial networks to create new synthetic images for data augmentation. may still not be enough for a given problem. If a dataset is very small, then a version augmented with rotation and mirroring etc. Geometric transformations, flipping, color modification, cropping, rotation, noise injection and random erasing are used to augment image in deep learning. The Augmentor library introduces elastic transforms in order to generate new synthetic images from a dataset in order to alleviate issues of scarcity.
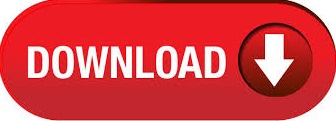